Introduction
Materials and Methods
Dataset
Artificial intelligence (AI) model
Selection of the ideal model
Results and Discussion
Conclusion
Introduction
Indoor farms have developed rapidly, as they contribute to producing fresh high-quality vegetables in buildings without being constrained by extreme climates or land limitations (Lee et al., 2013). In particular, plant factories have become popular as a solution to a dilemma in which there are three similar undesirable alternatives: (1) a shortage or unstable supply of food, (2) a shortage of resources, and (3) degradation of the environment (Kozai, 2018). Compared to greenhouse crop production, plant factories are energy efficient as greenhouse light is not regulated, and crop production can be affected by the time of day and by environmental changes (Kozai et al., 2019).
However, plant growth in plant factories is affected by internal and external factors, such as the nutrient supply and environmental conditions (Rizkiana et al., 2021). Therefore, diseases and disorders can occur more regularly if the conditions are not suitable for growing plants. Indoor-grown leafy green crops are frequently prone to tipburn, is a physiological disorder. Calcium (Ca) shortages in the developing leaves of the crop result in the bursting of laticifer cells due to inadequately developed cell walls. Tipburn manifests as visible necrosis in a young plant, developing on the leaves and possibly reducing the value of the harvest. It is known that Ca deficiency is the main cause of tipburn (Kaufmann, 2023). Ca is a crucial component of plant cell walls, and once fixed to the cell wall, it is immobile. Consequently, Ca cannot move between tissues with different Ca levels, and tissues often compete for the distribution of Ca delivered by the roots (Kubota et al., 2022). The leaf area is one component that affects the distribution of Ca, and the leaf Ca content is highly correlated with the cumulative transpiration rate. As immature leaves are smaller and have a lower transpiration rate than mature leaves, the drawing power of Ca from vascular tissue in immature leaves is low (Maruo and Johkan, 2020).
Early detection of tipburn is important for proper and timely treatment. However, the manual detection of tipburn is not an easy task because it requires skilled labor and considerable time for more precise identification of this condition. Machine learning with convolutional neural networks, more effective than manual detection, is commonly used as a solution to detect lettuce tipburn occurrence and non-occurrence (Thakur et al., 2022).
Experiments have proven that it is possible to identify tipburn symptoms with a high degree of accuracy using deep learning methods, and the early phases of tipburn that occur immediately before the leaf tips become black can be detected to prevent the commercial value of the crops from being compromised (Koakutsu, 2019). A wide range of neural network models is available and has been used in several studies. Different types of software can be used to analyze the images. However, different neural network models have different levels of accuracy (Mehrer et al., 2020). Therefore, it is necessary to identify an ideal model that can detect the disease more precisely.
Python is a powerful programming language that is easily read and understood. Systems have been developed with sets of algorithms using Python programming that can easily identify disease types (Xenakis et al., 2020). The input image provided by the operator undergoes various processing steps to distinguish the disease and the outcomes are returned to the user (Raut and Nage, 2019). Therefore, the Python programming language is commonly used in deep convolutional neural network processes (Kang et al., 2020; Rao et al., 2021).
In addition to Python, which is a programming language consisting of code, Orange 3 is an open-source machine-learning software that provides a visual interface for learning and data visualization (Ishak et al., 2020). Furthermore, it provides a user-friendly graphical interface that allows operators to work with data interactively, execute various data analytic tasks, and create machine-learning models without requiring advanced programming knowledge (Ratra and Gulia, 2020). Moreover, Orange 3 contains a comprehensive set of machine-learning algorithms and techniques that enable users to develop and assess models using the algorithms through a visual interface, providing users with the capability to combine a range of data processing, visualization, and modeling components to generate data analysis workflows (Demšar and Zupan, 2012).
New add-ons can be included in the Orange Canvas depending on requirements unless the essential widgets do not exist by default (Mohapatra and Swarnkar, 2021). The ‘Image analytics’ add-on must be installed for an image analysis, which consists of importing images, image viewer, image embedding, image grid, and saved image widgets. The ‘Import images’ widget is used to upload a folder containing the images to be analyzed. The ‘Image Embedding’ widget is used to continue with the standard machine-learning methods, which have trained embedders (neural network models) to conduct particular tasks. For example, the precision can differ for SqueezeNet, Inception v3, VGG-16, VGG-19, Painters, and DeepLoc (Demšar and Zupan, 2013). Further, to determine the model’s performance, two metrics, i.e., ‘precision’ and ‘recall,’ are used. Accuracy is determined from ‘precision’ while the effectiveness of the trained model in classifying all of the images is shown by the ‘recall’ value (Vaishnav and Rao, 2018). Moreover, the dataset’s images can be displayed by the ‘Image Viewer’ widget regardless of whether they are saved online or locally (Demšar and Zupan, 2013). The objective of this study was to find the most accurate neural network model for classifying leaves as either having tipburn and being healthy using the Python and Orange 3 software.
Materials and Methods
Dataset
Lettuce seeds were sown in trays containing polyurethane sponges. Seedling images were captured at every different stage, from seed germination to the transplanting stage, as shown in Fig. 1. After the lettuce plants were transplanted into the hydroponic system, individual plant images were captured for the analysis. In the hydroponics system, Hoagland nutrient solution was supplied and the electrical conductivity (EC) was set to 1.0 dS·m-1, with the pH maintained in the range of 5.5 to 6.0. In total, 447 plant images were captured using a mobile phone (Samsung Galaxy A73 5G, Korea). These images were classified into two categories: tipburn and healthy. Images representative of each category are shown in Fig. 2. From the total of 447 images, 375 images were classified as healthy while 72 images were sorted into the tipburn category. The total dataset was divided into two parts, with 357 images used for training and 90 images used for testing, to evaluate the accuracy of the neural network model.
Artificial intelligence (AI) model
Twenty-four different types of neural network models were evaluated using Python: DenseNet121, DenseNet169, DenseNet201, EfficientNet B0, EfficientNet B1, EfficientNet B2, EfficientNet B3, EfficientNet B4, EfficientNet B5, EfficientNet B6, EfficientNet B7, InceptionResNetV2, Inception V3, MobileNet, MobileNetV2, MobileNetV3Small, NASNetMobile, ResNet101, ResNet101V2, ResNet50, ResNet50V2, VGG 16, VGG19, and Xception, while Inception V3 was used in the Orange 3 program. To train all of the models, a transfer learning approach with a 224-pixel input resolution was used in Python, and two classes, 50 epochs, 32 batch sizes, the Adam optimizer, and a learning rate of 0.001 were applied. The Adam solver, the ReLU activation function, 100 neurons in the hidden layers, a maximum of 200 iterations in the neural network, and 0.0001 regularizations were applied in Orange 3. Python was used to write the code for detecting leaves showing tipburn. The workflow in Orange 3 is generated through widget connections (Fig. 3). The ‘Image analytics’ add-on was installed, as it is not included by default and is needed for the image analysis tasks.
Selection of the ideal model
Three performance evaluation metrics, i.e., precision, recall, and F1, were recorded to select the optimal neural network model and performance outcomes. Each metric is shown vertically, and the corresponding formulas are provided as (1), (2), and (3), respectively (Tripathi, 2021).
Precision represents the number of true positive predictive values obtained when positive labels were provided. Recall is similar to the true positive rate, describing the number of correctly labelled positive instances and sometimes referred to as the sensitivity (Bhatti et al., 2020). The F1 score, a metric that considers a combination of both Precision and Recall, is used to measure the overall performance of the model. True positive (TP) represents cases in which the true answer is predicted as true, whereas a false negative (FN) arises when the true answer predicted is false. Conversely, false positives (FP) indicate false answers predicted as true, while true negatives (TN) indicate false answers predicted as false (Tatbul et al., 2018).
The best model for this experiment was chosen based on its F1, accuracy, and recall values, which were the greatest among all other models and have values closer to one. Additionally, the TP, TN, FP, and FN numbers of images were used to determine the ideal AI model, as determined by the best performance matrix.
Results and Discussion
A machine-vision-guided plant sensing and monitoring system utilizing temporal, morphological, and color changes of plants has been used to identify calcium insufficiency in lettuce crops cultivated in greenhouse environments. This system can detect calcium-deficient lettuce plants a day earlier than human vision can sense visual stress (Story et al., 2010). Several studies have attempted to detect lettuce tipburn using AI models under different conditions. Hamidon and Ahamed (2022) suggested that deep-learning algorithms can be used to identify tipburn in lettuce cultivated in indoor farms under various lighting conditions with a reasonable overall accuracy level. The present study was also conducted in a closed-type plant factory, and healthy and tipburn images were taken.
Datasets are the most crucial component of deep-learning algorithms. Both the input quality and quantity are very important when generating an ideal model and a highly efficient system. In this experiment, numerous images were taken (447 images). All tipburn and healthy leaves images were collected from several batches of plant growth, starting from the date of transplantation and extending to the harvesting stage in a plant factory. Fig. 4 shows the development of tipburn in lettuce plants during each stage. Early stages of tipburn, as well as the severe stages of symptoms, were captured from the date of transplanting to the harvesting stage to train AI models using tipburn symptoms at each growth stage ultimately to assist farmers in identifying early tipburn stages. Additionally, tipburn symptoms are typically difficult to distinguish in the early stages of plant development from other disease symptoms that may arise from microorganisms due to similar symptoms. Consequently, farmers can use preventive measures in their future cultivations depending on their requirements if they can more accurately diagnose tipburn symptoms in the early stages utilizing AI models. Therefore, this study is crucial because it presents a method for the more precise identification of lettuce tipburn symptoms with AI models that were trained using the images taken in each stage of tipburn development over time (Figs. 2 and 4). However, generating a tipburn dataset in an indoor system from batch to batch is a highly complicated and protracted task because in plant factories, plants are stacked on top of one another, closely packed, and grown indoors under artificial lighting. Using human labor, it is challenging to observe individual plants in such dense growth conditions. Consequently, it is crucial to have a camera system capable of automatically detecting tipburn symptoms, which would be especially important for large-scale lettuce production. Moreover, using AI models is more accurate given the possibility of human error. Furthermore, it may be difficult and limited to find specialists who can evaluate these issues, especially given the complex and condensed development conditions of an indoor farming system. For these reasons, the most effective way is to use computer or machine vision systems to detect tipburn automatically (Shoaib et al., 2023). Several studies have developed low-cost AI-based technologies with the highest accuracy. Examples include studies conducted by Gozzovelli et al. (2021), Kirwan et al. (2023), Chen et al. (2023), and Dasgupta et al. (2020), among others. Therefore, it is cost-effective and more precise to use AI models for large-scale cultivation rather than using human labor. However, an imbalanced dataset may contain bias, resulting in models lacking sufficient data to learn and causing the low detection of tipburn in lettuce. Therefore, more images were collected and labeled appropriately to enhance the accuracy of tipburn detection. To overcome biased results while training the models, data augmentation was used to increase the similarity in the dataset. According to Candemir et al. (2021), it is crucial to use data augmentation, which enables one to increase the number of training samples in an artificial manner. This enhances convergence, minimizes overfitting, and improves the performance of the model’s predictions. An uneven class distribution within the dataset or inadequate training data can be avoided by data augmentation (Mikołajczyk and Grochowski, 2018), and generating novel iterations of an existing dataset and having models acquire different iterations of similar objects or situations in which the initial dataset is insufficient or small for training model data augmentation would be beneficial (Singh et al., 2020). Further, by adding numerous modifications to the original images, data augmentation can assist in enhancing the model’s robustness and generalization capabilities and thus generate better results (Shi et al., 2022).
As shown in Tables 1 and 2, different types of AI models were used for tipburn detection with two different software packages; Python and Orange 3. Python is a commonly used programming language for machine learning. It is the ideal option for creating AI models because libraries and open-source tools are readily available. The extensive library also helps to reduce the workload (Saabith et al., 2020). In this study, five AI models, MobileNetV2, DenseNet169, DenseNet121, InceptionResNetV2, and MobileNet, which were analyzed with Python showed the highest accuracy compared to the Inception V3 model used in the Orange 3 program. Among the models, MobileNetV2 showed the highest accuracy (0.933), followed by DenseNet169 (0.911), DenseNet121 (0.900), InceptionResNetV2 (0.900), and MobileNet (0.900). MobileNetV2 was used by Dong et al. (2020) for image classification to compare MobileNetV1 and MobilNetV2. Their results showed high accuracy rates in the MobileNetV2 model on image classification tasks compared with MobileNetV1, consistent with our study. An earlier version of MobileNetV1 was used as the core structure for MobileNetV2. In addition to addressing the issue of information loss in nonlinear layers in convolution blocks using linear bottlenecks, MobileNetV2 introduces a new structure called inverted residuals to preserve information. Depth-wise separable convolution (DSC) is a technique used by MobileNetV2 for portability (Dong et al., 2020). An intriguing connectivity pattern is found in DenseNet, where every layer in a dense block is connected to every other layer. Because all layers in this instance have access to feature maps from earlier layers, there is a strong incentive for excessive feature reuse. The model is hence less prone to overfitting and is more compact. Additionally, implicit deep supervision is provided by the shortcut paths, which allow the loss function to supervise each layer directly. With all of these advantageous characteristics, DenseNet is a perfect match for per-pixel prediction issues (Zhu and Newsam, 2017). In this study, three DenseNet AI models were used, DenseNet169, DenseNet121, and DenseNet201, with corresponding accuracy rates of 0.9111, 0.9000, and 0.8889. However, the accuracy rates of DenseNet121, InceptionResNetV2, and MobileNet models (0.9111) were similar. Moreover, eight EfficientNet models were used, from B0 to B7 in this study, and these results showed that those models have low accuracy for lettuce tipburn detection compared to other models (Table 1). A similar study was conducted to identify diseases in papaya by Hridoy and Tuli (2021), who suggested that the EfficientNet B5, B7, and B6 models had the highest accuracy rates. Tan and Le in 2019 showed that the accuracy increases with the model number. However, in this study, the accuracy rates of all EfficientNet models (B0 to B7) were identical (0.8222). The ResNet152V2 model had the lowest accuracy among all models analyzed by Python, and also the accuracy was lower than the Inception V3 model (0.862) analyzed by the Orange 3 software.
Table 1.
Accuracy rate of each ai model analyzed using python
The Inception V3 model in the Orange 3 software was used in a performance comparison with the Python AI models. The Orange data mining tool is visual programming software that offers versatility by combining model training, testing, data preprocessing, and visualization into a single software package for easy operation for users (Vaishnav and Rao, 2018; Ratra and Gulia, 2020). An interactive environment with a high level of functionality can be customized through visual programming and enable the creation of workflows customized for specific problems (Godec et al., 2019). In this study, the workflow was generated for the purpose of an image analysis, as shown in Fig. 3, and for this, the image analytics add-on was installed in the Orange 3 software. Inception V3 was selected as the AI model. Two separate paths were used for training and testing images. In this experiment, as shown in Table 2, three performance metrics were recorded to measure the precision of the Inception V3 model in the Orange 3 program. When predicted and actual values are similar, the total true positive scores are indicated by the Precision score, While Recall indicates the number of true positive instances among all positive instances. The F1 score, a metric that considers a combination of both Precision and Recall, is used to measure the overall performance of the model (Vaishnav and Rao, 2018). In this experiment, the precision, recall, and F1 scores of the Inception V3 model that was analyzed by the Orange 3 program were 0.862, 0.873, and 0.865, respectively. However, the precision of the Orange 3 Inception V3 model was lower than those of the models evaluated by Python, in this case MobileNetV2, DenseNet169, DenseNet121, InceptionResNetV2, MobileNet, DenseNet201, VGG19, ResNet50V2, and VGG 16. Though the accuracy of the Orange 3 AI model was low compared to the Python models, it was at a satisfactory level, as it exceeded 80%. Inception V3 is a convolution neural network-based model from the Inception family that uses several techniques, such as 7x7 factorized convolution, label smoothing, and an auxiliary classifier to enhance/outperform previous models from the Inception family (Szegedy et al., 2016). Inception V3 was applied by Qian et al. (2019) to measure the accuracy of classification results according to the recording precision, recall rate, and F1 score; the model showed a significant effect on a dataset of fresh tea leaves. Similarly in our experiment involving the use of the Inception V3 model in the Orange 3 program, the accuracy of the model was satisfactory to classify tipburn on lettuce leaves. Further, using Orange 3 is much easier, as it provides a graphical interface with which to create workflows (Fig. 3).
Table 2.
Precision, recall and F1 score of the Inception V3 models as analyzed by Orange 3
Model | Precision | Recall | F1- score |
Inception V3 | 0.862 | 0.873 | 0.865 |
The slightly lower accuracy of the Inception V3 model in the Orange 3 program compared to that of the Python MobileNetV2 model can stem from different factors. Different algorithms in the Orange 3 program can be used for a comparison, such as logistic regression, neural networks, k-nearest neighbors, decision trees, random forests, and naïve Bayes. A neural network algorithm was used in this study. Neural networks, which are deep learning algorithms, use an error estimation approach that utilizes weight adjustments to generate a network and attain a satisfactory judgment. In a neural network, feature extraction from a dataset and classification occur simultaneously (Vaishnav and Rao, 2018). However, the accuracy can change depending on the type of algorithm used. In addition, it is possible to produce varying results with different datasets. However, in this experiment, the same dataset was used to analyze the accuracy of the models with Python and Orange 3. The accuracy of the model can also vary depending on the type of activation function used (Asriny et al., 2020). The ReLU activation function was used in this study. One recent study (Ramachandran et al., 2017) found that ReLU is the most popular and effective activation function. However, they suggested that deep network training dynamics and task performance are significantly impacted by the choice of activation functions. Therefore, in this study, the type of algorithm and the activation function used may have been factors in the slightly lower precision of the Inception V3 model compared to that of the Python MobileNetV2 model. It will be important to test different algorithms and activation functions in future studies to obtain more accurate results using Orange 3.
The performance of the supervised learning algorithm is indicated by a confusion matrix (also known as an error matrix or a contingency table), where each column represents the instances in a predicted class and each row represents the actual class instances. Therefore, a confusion matrix can assess the error or difference between actual and predicted values. The off-diagonal elements of the confusion matrix determine the error in the prediction, whereas the diagonal elements determine the accuracy of the prediction. Using the Orange 3 program, it was easy to visualize the ‘confusion matrix’ using the corresponding widget (Fig. 5). When considering the confusion matrix obtained using the Orange 3 program, there were some cases of incorrect predictions using the Inception V3 model, in which healthy leaves were classified as those showing tipburn and leaves showing tipburn were predicted as healthy leaves, as shown in the off-diagonal cells. For an additional interpretation, the confusion matrix can be used to compute the number of True Positive (TP), True Negative (TN), False Positive (FP), and False Negative (FN) images (Fig. 6). The numbers of TP, TN, FP, and FN images were calculated, as shown in Fig. 7. However, the precision of the Inception V3 model obtained by the Orange 3 program was considerably higher than that of Python’s Inception V3 model (Tables 1 and 2). The Orange 3 Inception V3 model and the Python Inception V3 model’s accuracy rates were 0.862 and 0.833, respectively. However, in future studies, it would be better to analyze the accuracy rates of every model available in Orange 3, as the performance can differ depending on the AI model.
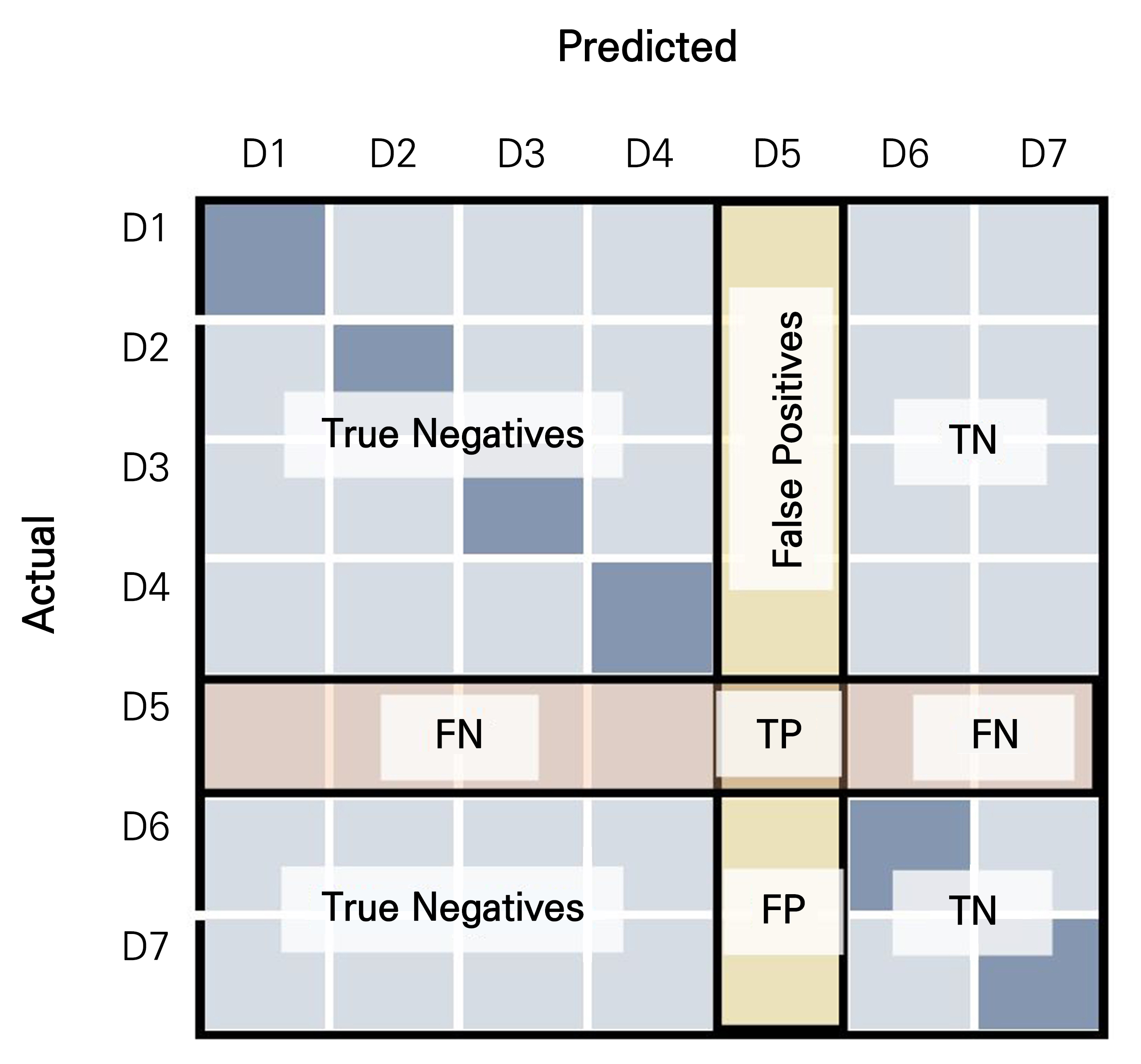
Fig. 6.
Process of sorting images from a confusion matrix that corresponds to True Positive (TP), True Negative (TN), False Positive (FP), and False Negative (FN) for a specific image category (with D5 chosen in this figure). Seven image categories are shown in this figure for a clear explanation. In this study, only two image categories, healthy and tipburn, were used. A study by Patro and Patra (2014) was referred to during the creation of this figure.
It is crucial to consider the possibility of AI models for real-world applications. Singh (2018) discussed several real-world applications of AI models. Farmers can use smartphone mobile apps that allow a quick and correct identification of diseases and can find treatments by photographing diseased plant parts. Real-time diagnosis is possible with the most recent artificial intelligence (AI) algorithms for cloud-based image processing. To improve its accuracy, the AI model continuously learns from user-uploaded photographs and expert advice. Farmers can also communicate with local experts via the platform. Shrimali (2021) developed a free, user-friendly, and widely accessible mobile application for diagnosing plant diseases using MobileNetV2. This program includes treatment stages, typical symptoms, and connections to recommended cures for diseases. Therefore, real-time crop disease diagnosis is possible based on a trained MobileNetV2 as an innovative and accessible tool for crop disease control that provides farmers with a free service to promote environmentally sustainable agriculture and increase food security. In this study, MobileNetV2 had the highest accuracy rates among the models analyzed by Python. Moreover, Inception V3, used in the analysis by Orange 3, had a considerably high accuracy rate. It is more convenient for farmers to use Orange 3 software, as it has a graphical interface that facilitates the simple operation of real-world applications without expert knowledge, meaning that it is now possible to use AI models for real-world lettuce tipburn identification easily via farmers’ smartphones, especially considering that this method has been shown in previous research to be a low-cost approach that is most accurate and highly feasible with real-world applications.
Conclusion
Early detection of tipburn is beneficial for growers to manipulate the controlled environment parameters to improve the freshness of lettuce grown in plant factories. In Python, MobileNetV2 was most accurate (0.933) followed by DenseNet169 (0.911), DenseNet121 (0.900), InceptionResNetV2 (0.900), and MobileNet (0.900). The precision rate of the Orange 3 Inception V3 model was 0.862. Though the accuracy of the Orange 3 AI model was low compared to the Python models, it was at a satisfactory level in that it exceeded 80%.